Notice
Recent Posts
Recent Comments
Link
일 | 월 | 화 | 수 | 목 | 금 | 토 |
---|---|---|---|---|---|---|
1 | 2 | 3 | 4 | 5 | ||
6 | 7 | 8 | 9 | 10 | 11 | 12 |
13 | 14 | 15 | 16 | 17 | 18 | 19 |
20 | 21 | 22 | 23 | 24 | 25 | 26 |
27 | 28 | 29 | 30 |
Tags
- Reinforcement Learning
- canny edge detection
- dfs
- real-time object detection
- image processing
- C++
- opencv
- BFS
- 강화학습
- machine learning
- CNN
- YoLO
- MySQL
- MinHeap
- object detection
- eecs 498
- DP
- One-Stage Detector
- 딥러닝
- r-cnn
- 머신러닝
- Mask Processing
- two-stage detector
- AlexNet
- dynamic programming
- Python
- 백준
- LSTM
- 그래프 이론
- deep learning
Archives
- Today
- Total
목록ablation study (1)
JINWOOJUNG
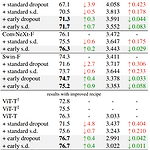
4. Approach모델의 underfitting, overfitting regime(상태)을 결정하는 기준은 다음과 같이 정의된다.Overfitting모델이 표준 dropout에서 더 좋은 일반화 성능을 보이는 경우표준 dropout은 training 전 과정에서 dropout 적용Underfitting모델이 dropout이 없을 때 더 좋은 성능을 보이는 경우본 논문에서는 각각의 상태에 따른 서로 다른 dropout 적용 방법을 제안한다. $\quad$Underfitting : early dropout Underfitting 상태의 모델은 dropout을 사용하지 않는 것을 기본 설정으로 한다. 이때, 모델이 training data에 더 잘 맞춰지도록 하기 위한 early dropout을 제안한다...
딥러닝/논문
2024. 12. 31. 15:36