일 | 월 | 화 | 수 | 목 | 금 | 토 |
---|---|---|---|---|---|---|
1 | 2 | 3 | ||||
4 | 5 | 6 | 7 | 8 | 9 | 10 |
11 | 12 | 13 | 14 | 15 | 16 | 17 |
18 | 19 | 20 | 21 | 22 | 23 | 24 |
25 | 26 | 27 | 28 | 29 | 30 | 31 |
- Reinforcement Learning
- r-cnn
- deep learning
- ubuntu
- AlexNet
- Mask Processing
- 그래프 이론
- eecs 498
- NLP
- One-Stage Detector
- dynamic programming
- Python
- 딥러닝
- YoLO
- MySQL
- 백준
- dfs
- DP
- two-stage detector
- C++
- CNN
- MinHeap
- 머신러닝
- LSTM
- BFS
- image processing
- machine learning
- opencv
- 강화학습
- real-time object detection
- Today
- Total
목록VGG (2)
JINWOOJUNG
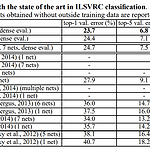
https://jinwoo-jung.tistory.com/133 Very Deep Convolutional Networks for Large-Scale Image Recognition...(1)Research Paperhttps://arxiv.org/abs/1409.1556https://papers.nips.cc/paper_files/paper/2012/hash/c399862d3b9d6b76c8436e924a68c45b-Abstract.htmlhttps://www.researchgate.net/publication/220812758_Flexible_High_Performance_Convolutional_Neural_Networks_for_Imajinwoo-jung.com4. Classification E..
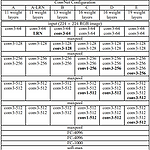
Research Paperhttps://arxiv.org/abs/1409.1556https://papers.nips.cc/paper_files/paper/2012/hash/c399862d3b9d6b76c8436e924a68c45b-Abstract.htmlhttps://www.researchgate.net/publication/220812758_Flexible_High_Performance_Convolutional_Neural_Networks_for_Image_Classification0. Abstract본 논문에서는 Convolutional Network의 Depth에 따른 성능을 분석한다. 3x3의 작은 Convolutional Filter를 사용하여 Network의 Depth를 16~19까지 증가시킴..